At Auror, we’re always looking at how we can transform the way retailers report, solve, and prevent crime in stores. In the last few months alone, we’ve made over 300 improvements to our platform to support our retail partners to reduce crime. We’re currently working on some big projects that we believe will be game-changers for the industry (get an invite to our upcoming webinars on these projects here).

On top of all this, we decided to break away from our routine and spend a week experimenting with some emerging technologies and out of the box ideas we thought could help our retail partners reduce crime in their stores. We called it “Innovation Week” and despite this not being a particularly innovative name, we’re excited to share some of the ideas that came out of this.
How did it work?
- Ideas were proposed in a Slack channel to get feedback from everyone in the company on whether it might have value to retailers, or where an idea could be used in a different way to have greater impact.
- Our software engineers then chose which ideas they wanted to work on for just 5 days (1-3 engineers per idea), with designers, testers, and product managers available to support where needed. There was a lot of collaboration happening throughout the week to answer questions, validate ideas, and share progress.
- At the end of the week the groups presented what they’d built, why they’d chosen to work on that particular idea, and gave a live demo of it working.
We ended up with nine functioning prototypes by the end of the week. These ranged from reporting incidents by voice to predicting hot products by region. We were excited to see how much time had been invested in prototyping practical applications for new and emerging tech like AI, Machine Learning, and Analytics.
The Experiments
We’re excited to share four of the highlights that came out of the 5 days of prototyping. Imagine how these innovations could empower your organization to reduce crime in your stores.
Disclaimer: the focus was on building functional prototypes and workflows with technologies with very little focus was put on the user interface of our prototypes.
Reporting a crime event through speech
Goal:
To be able to report an incident through speech on a mobile device and use natural language processing to understand what has been said and complete a draft incident.
Technology used:
Natural language processing and Microsoft Azure’s text analytics
What we prototyped:
We were able to capture speech using Siri or Google Assistant with surprising accuracy. Microsoft Azure’s Text Analytics service was then used to pull out relevant keywords from the text and Natural Language Processing applied context to recognise which aspects of the speech related to an offender, a vehicle, or a product. These were mapped to the relevant event fields and automatically populated a draft event report.
This would allow for capturing information at the time of the event from anywhere in the store and automatically create a draft event to complete when ready.
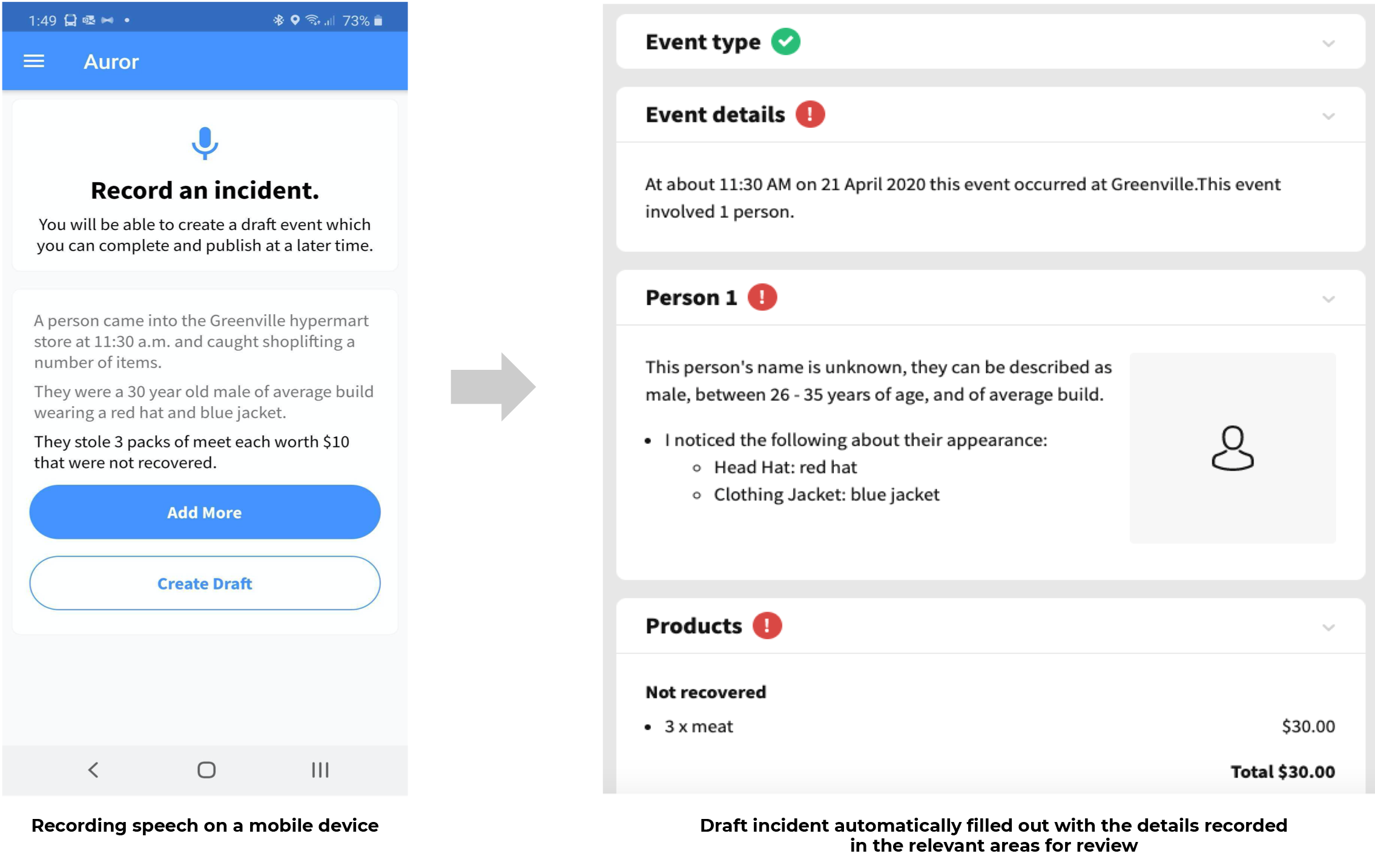
Takeaway for Asset Protection Leaders
Imagine the productivity impact for store operations if they could complete a detailed crime report on the shop floor in under a minute?! They could work interchangeably between mobile and desktop devices to report accurate, detailed incidents quickly.
Using computer vision to redact unrelated parties from images
Goal:
To automatically redact the faces of anyone in that image other than the subject in question and save a face shot for each subject.
Technology used:
Image recognition with Microsoft Azure cognitive services and computer vision
What we prototyped:
We were able to automatically redact the faces of these people to clearly identify the subject and protect the privacy of uninvolved people around them.

Takeaway for AP Leaders:
Use technology to improve the ease of compliance for end users and ensure your organization is not breaching privacy legislation.
Using computer vision to suggest matches
Goal:
To automatically identify if a person of interest has already been involved in a previous incident at your organization and suggest this link.
Technology used:
Image recognition with Microsoft Azure cognitive services and computer vision
What we prototyped:
We were able to check for a face in an uploaded image against existing persons of interest from previous incidents. If there was a high degree of confidence in the match, it will let the incident reporter connect the dots faster on repeat offending.
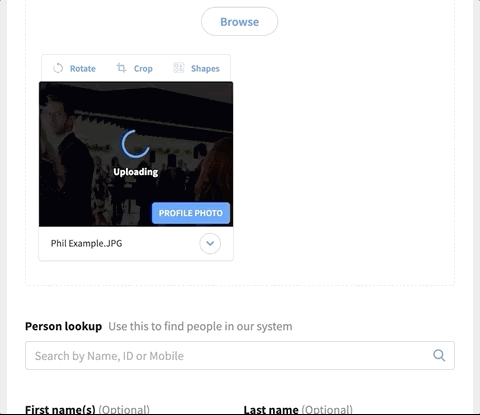
Takeaway for AP Leaders
Our retail partners are already reaping the benefits of having an image first platform for case management in terms of connecting the dots. With 10% of offenders responsible for 50% of the total value of incidents. Imagine when you can supercharge the linking of criminal activity across your network of stores with computer vision.
Using store maps to track spatial patterns in crime
Goal:
Understand the spatial patterns of crime within stores to help asset protection teams deploy their limited physical assets and measures of control as effectively as possible.
Technology used:
Data analysis, heat mapping, spatial mapping
What we prototyped:
We experimented with adding store maps and plotting where key crime event milestones (like entry, selection, concealment, apprehension, exit) happened for an event. We were then able to use that data to show an aggregated view to identify patterns and highlight the highest risk areas in stores.


Takeaway for AP Leaders
At an incident level this information may be useful from a prosecutorial perspective and at an aggregate level could help drive data-driven decisions around asset protection measures needed at that location.
Real time insights into crime trends impacting your stores
Goal:
Help retailers reduce loss by highlighting trends in real-time that could inform proactive decision-making to reduce loss.
Technology used:
Data analysis, anomaly detection, and machine learning
What we prototyped:
We prototyped a service that could consume historical data, train a machine learning model, and then detect trends in real-time. For the purposes of the prototype we focused on products targeted by region.

Takeaway for AP Leaders
This is a small glimpse into a future where smart systems continuously analyse data to produce insights which inform real-time data driven decisions. This will mean less of an onus on your organization and team to go digging into the data and being able to invest that time into making better decisions to reduce loss.
What’s next?
It was incredible to see what the team was able to achieve in just 5 days! We shared some of these live demos in recent webinars and based on feedback from attendees we’re excited about prioritising some of these features to help make retail crime reporting faster and more accurate - enabling AP teams to drive far more outcomes from intelligence.